Motivation
Shiny is light-weighted web application, that seamlessly integrated with R. By using shiny, R users can quickly build prototype data product from their existing R models or analysis. In my experience of working with business users, they usually prefer a unified portal, where they can find all analytical solutions provided by data team. Developing a unified shiny application is more preferable, compared with many stand-alone small shiny apps, because the end users don’t have to bookmark the URLs for all applications and are able to access all apps with single login action. It helps to standardize the style and format as well, but on the other hand, it will be more difficult to code and maintain, especially using the default single app.R
file structure. Therefore, I’m working on an extendable file structure to build the unified shiny portal.
Prerequisite
Packages
- shiny: use
navbarPage
to assemble different shiny apps.
- shinyjs: add powerful JavaScript capability to shiny, without prior knowledge of JavaScript.
- glue: advanced version of
paste
, useful to format strings.
HTML
shiny already wraps common HTML tags into shiny tags
object. Knowing common HTML tags, such as
- division tag:
div, tags$div()
- paragraph tag:
p, tags$p()
- thematic break tag:
hr, tags$hr()
will be sufficient for this post.
CSS
CSS controls the style of HTML elements by Class. This is preferable to create standardized web application, with no harm to your shiny app. CSS formatting file is located under ./www
folder for shiny.
Shiny File Structure
In order to make it easy to unit test each individual shiny app, I created directories for each shiny app, where I also have an app.R
for testing purpose.
app.R
in sub-folder: used for unit testing of this individual shiny app.
app.R
in main-folder: used for integration testing of unified shiny app.
tab.R
in sub-folder: used to create a list, that wraps the ui
and server
of this shiny. After passing the unit test, it can be directly import to main shiny, for integration testing.
global.R
: used to define global settings, such as database connections, login credentials, page headers/footers.
main.css
in www-folder: used to define the style of shiny app, by pointing to theme
argument in navbarPage
. In shiny, the images, css files or JavaScript codes are all located under www
folder.
├── 00_tab_login # sub directory for login page
│ ├── app.R # shiny app for unit testing
│ └── tab.R # define a list, wrapping ui and server
├── 01_tab_dashboard_01 # sub directory for dashboard 01
│ ├── app.R
│ └── tab.R
├── 02_tab_dashboard_02 # sub directory for dashboard 02
│ ├── app.R
│ └── tab.R
├── app.R # main shiny app
├── global.R # define global settings
├── readme.md
└── www
└── main.css # shiny css file
4 directories, 10 files
Using this file structure, I can easily add/remove certain shiny tab, without affecting other components.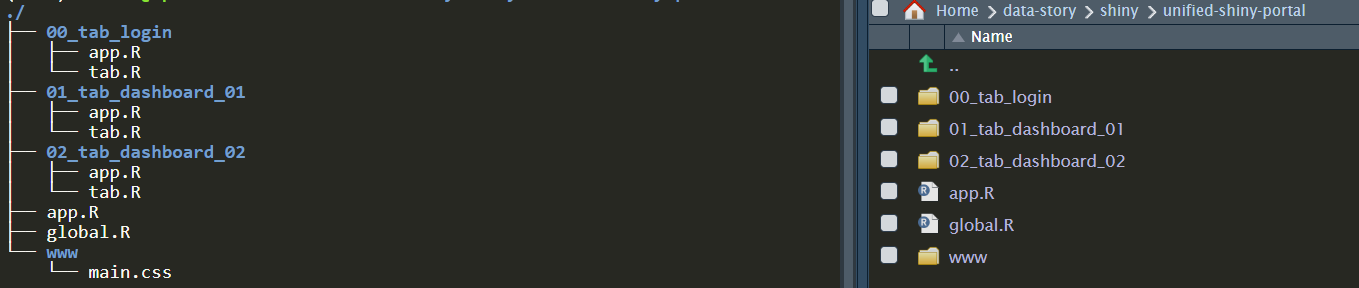
Login Page
First of all, let’s create a login page to secure our unified shiny app. shiny::modalDialog
is used to create the pop-up dialog box, which stops the user accessing the content of shiny app. We just need to add one observeEvent
on login button to validate the user input. If user input is correct, remove the dialog box and show the welcome message, otherwise keep the dialog box and show the warning message.
Login Page – UI
The ui
component is a tabPanel
object, which just contains a hidden welcome message in a div
tag.
id
is set for shinyjs
to locate JavaScript actions on it.
class
is set for CSS
to identify the formatting styles.
login_dialog
is another ui for the login dialog box, which allows user to input username and password. This UI will be controlled by server
.
# define a list to wrap ui/server for login tab
tab_login <- list()
# define ui as tabPanel,
# so that it can be easily added to navbarPage of main shiny program
tab_login$ui <- tabPanel(
title = 'Login', # name of the tab
# hide the welcome message at the first place
shinyjs::hidden(tags$div(
id = 'tab_login.welcome_div',
class = 'login-text',
textOutput('tab_login.welcome_text', container = tags$h2))
)
)
# define the ui of the login dialog box
# will used later in server part
login_dialog <- modalDialog(
title = 'Login to continue',
footer = actionButton('tab_login.login','Login'),
textInput('tab_login.username','Username'),
passwordInput('tab_login.password','Password'),
tags$div(class = 'warn-text',textOutput('tab_login.login_msg'))
)
Login Page – Server
server
component is a function of input
,output
or session
(usually optional), that takes input
generated by user and render the changes to output
. (Note: user.access
is a R list, generated from global.R
)
# define the backend of login tab
tab_login$server <- function(input, output) {
# show login dialog box when initiated
showModal(login_dialog)
observeEvent(input$tab_login.login, {
username <- input$tab_login.username
password <- input$tab_login.password
# validate login credentials
if(username %in% names(user.access)) {
if(password == user.access[[username]]) {
# succesfully log in
removeModal() # remove login dialog
output$tab_login.welcome_text <- renderText(glue('welcome, {username}'))
shinyjs::show('tab_login.welcome_div') # show welcome message
} else {
# password incorrect, show the warning message
# warning message disappear in 1 sec
output$tab_login.login_msg <- renderText('Incorrect Password')
shinyjs::show('tab_login.login_msg')
shinyjs::delay(1000, hide('tab_login.login_msg'))
}
} else {
# username not found, show the warning message
# warning message disappear in 1 sec
output$tab_login.login_msg <- renderText('Username Not Found')
shinyjs::show('tab_login.login_msg')
shinyjs::delay(1000, hide('tab_login.login_msg'))
}
})
}
Component Testing
After defined ui
and server
in tab.R
, we now are ready to do the unit testing for shiny login page.
- set the working directory to the sub-folder.
setwd('./00_tab_login')
- create
app.R
as follow, by assigning the ui
and server
from tab.R
library(shiny)
library(shinyjs)
library(glue)
source('tab.R') # load tab_login ui/server
ui <- navbarPage(
title = 'Login',
selected = 'Login',
useShinyjs(), # initiate javascript
tab_login$ui # append defined ui
)
server <- tab_login$server # assign defined server
shinyApp(ui = ui, server = server)
The tested login page
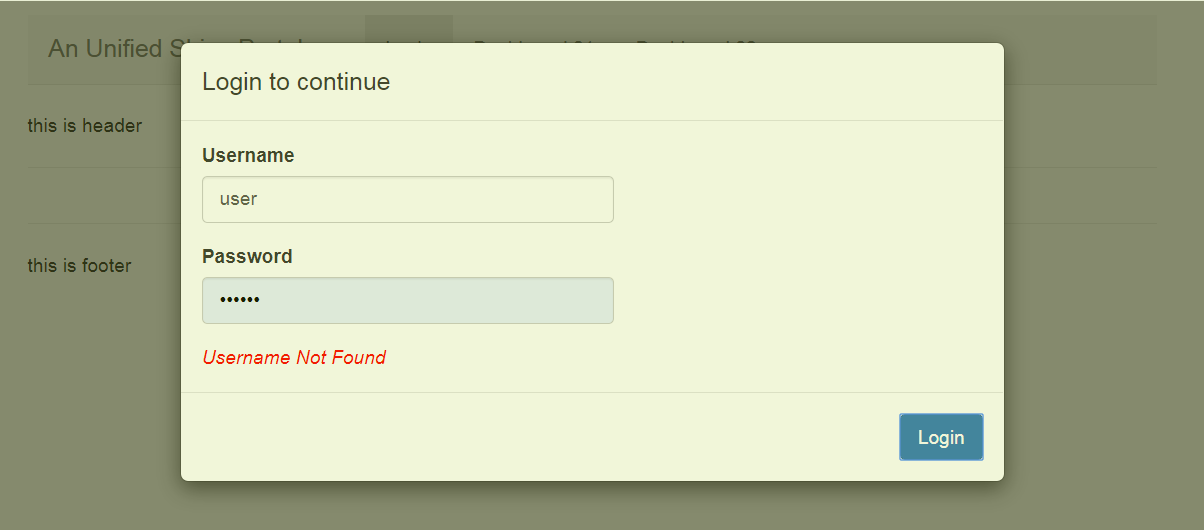
When successfully login, the page should be shown like this:
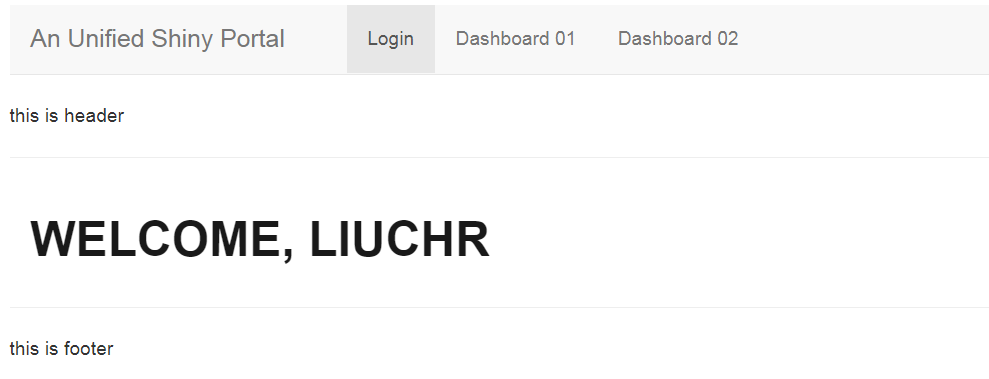
Integration Testing
After login page is tested, I also created two dummy dashboards dashboard 01
and dashboard 02
.
Similar to 00_tab_login
, 01_tab_dashboard_01
and 02_tab_dashboard_02
are structured with tab.R
and app.R
.
01_tab_dashboard_01
: histgram of normal distribution with sample size N (code details)
02_tab_dashboard_02
: estimation of pi using monte carlo simulation (code details)
After component testing of each shiny app is done, we can now put all shiny tabs together to form the unified shiny portal.
library(shiny)
library(shinyjs)
library(glue)
# load global parameters (DB connections, login credentials, etc)
source('global.R')
# load ui/server from each tab
source('./00_tab_login/tab.R')
source('./01_tab_dashboard_01/tab.R')
source('./02_tab_dashboard_02/tab.R')
app.title <- 'A Unified Shiny Portal'
ui <- navbarPage(
title = app.title,
id = 'tabs',
selected = 'Login',
theme = 'main.css', # defined in www/main.css
header = header, # defined in global.R
footer = footer, # defined in global.R
# initiate javascript
useShinyjs(),
# ui for login page
tab_login$ui,
# ui for dashboard_01
tab_01$ui,
# ui for dashboard_02
tab_02$ui
)
server <- function(input, output) {
# load login page server
tab_login$server(input, output)
# load server of dashboard_01
tab_01$server(input, output)
# load server of dashboard_02
tab_02$server(input, output)
}
# Run the application
shinyApp(ui = ui, server = server)
the final dashboard 01 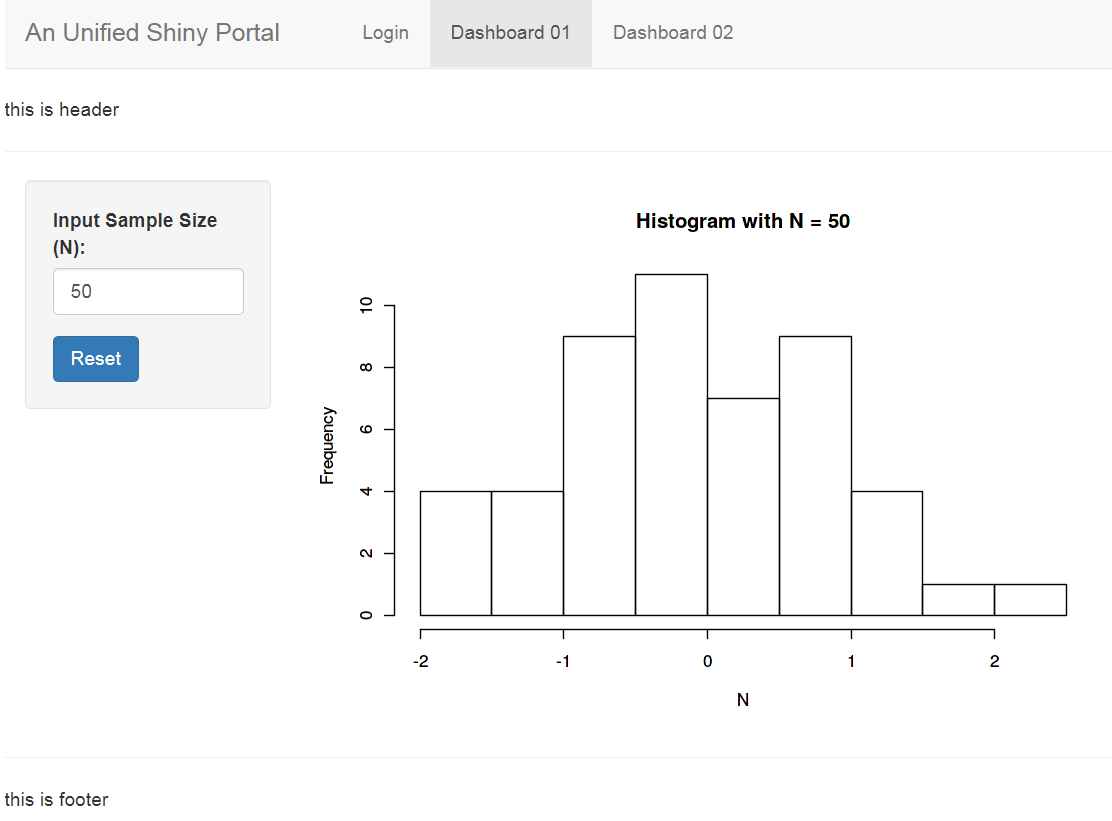
the final dashboard 02 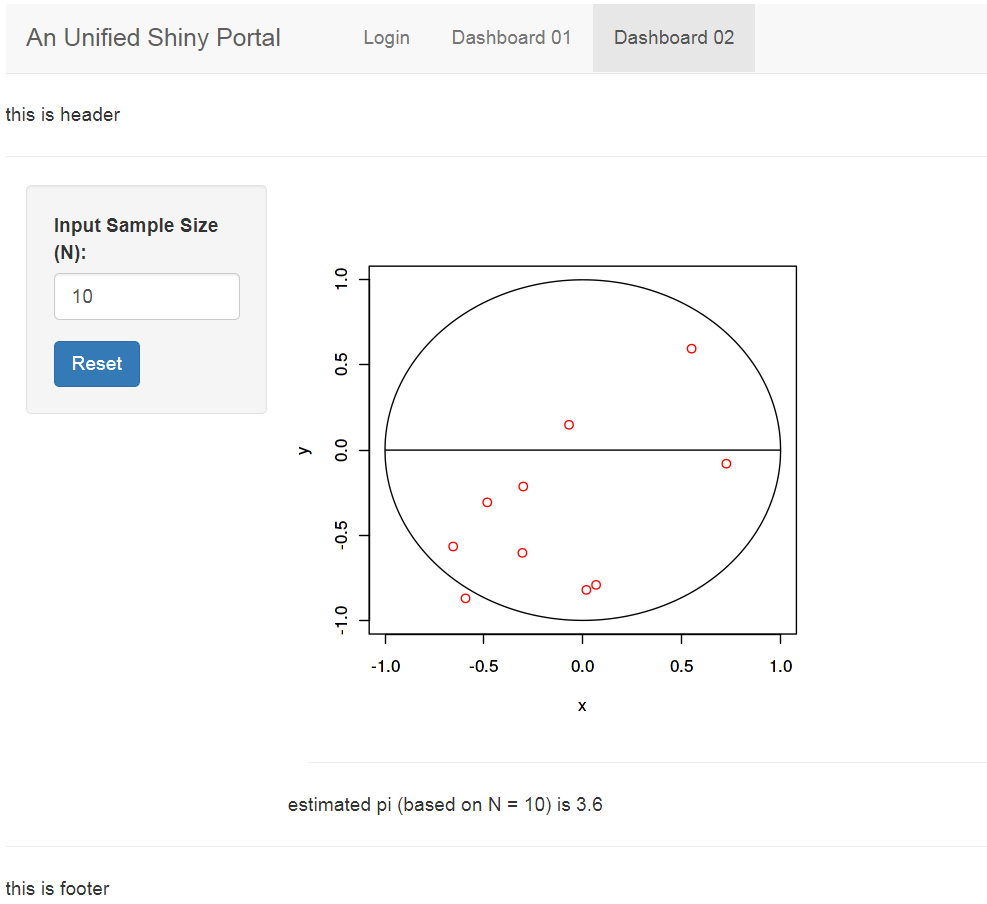
Conclusion
In summary, in order to build a unified shiny portal:
Login Page
- use
shiny::modalDialog
to create dialog box for login page
- use
shiny::showModal
,shiny::removeModal
,shinyjs
to control the dialog box.
Extendable Shiny Tabs
- create separate folders for shiny tabs, which consists of
tab.R
defining ui
and server
and app.R
defining code for component testing.
- load pairs of
ui
and server
from each tab in app.R
of main shiny page
- test, test and test
Complete Unified Shiny Portal
login credential ( username: liuchr, password: 123456 )
1.277673
103.848234